How can analytics enhance risk management decision-making
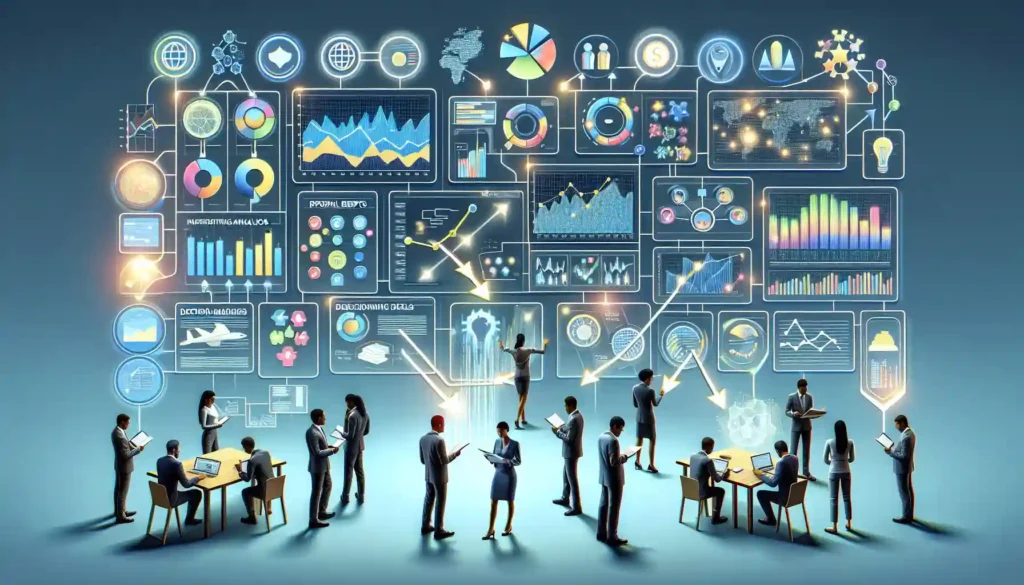
Analytics in risk management has emerged as a crucial discipline that enables businesses to navigate the complexities of potential threats effectively. By harnessing the power of data analytics, organizations are now able to make informed decisions that enhance their risk management strategies. This data-driven approach not only reduces vulnerabilities but also positions firms to capitalize on opportunities, ensuring a competitive edge in their respective markets.
In today’s dynamic business environment, where uncertainties abound, the integration of risk management and analytics has become more essential than ever. Companies are embracing technology-driven solutions that leverage big data, artificial intelligence, and machine learning to uncover insights that traditional methods often miss. The result is a transformative impact on risk management decision-making processes, making them more robust, proactive, and responsive to emerging threats.
- Understanding the Role of Analytics in Risk Management Decision-Making
- Key Stages of Risk Management Enhanced by Analytics
- Leveraging AI for Proactive Risk Monitoring
- Integrating Risk Libraries for Comprehensive Risk Analysis
- The Benefits of Structured Risk Analytics Solutions
- Aligning Risk Management Strategies with Business Objectives
- Improved Communication through Analytics in Risk Management
- Real-World Examples of Successful Risk Management Analytics
- Overcoming Challenges in Implementing Risk Analytics
- Future Trends in Analytics for Risk Management Decision-Making
Understanding the Role of Analytics in Risk Management Decision-Making
The role of analytics in risk management is pivotal for organizations striving to maintain resilience in the face of potential threats. Analytics enhances the risk management framework by providing insightful data that informs decision-makers about various risk-related aspects, such as likelihood, impact, and overall risk exposure. By employing predictive analytics, businesses can identify potential risks before they materialize, enabling them to act preemptively.
Incorporating analytics means shifting from a reactive stance to a proactive approach in managing risks. For example, when analyzing historical data patterns, organizations can forecast future risks and prepare strategies to mitigate them effectively. This approach not only fortifies the risk management strategy but also supports informed decision-making, aligning risk assessment with organizational objectives.
The Importance of Data-Driven Insights in Identifying Risks
Data-driven insights are invaluable when it comes to identifying risks that may otherwise remain invisible through traditional methods. By using advanced analytics, organizations can sift through vast amounts of data, seeking anomalies and patterns that indicate potential risks. Here are several ways this process plays a critical role:
- Enhanced Visibility: Analytics allows firms to gain complete visibility into their operational landscape and financial health, enabling risk managers to identify weak spots more effectively.
- Real-Time Analysis: Continuous data monitoring provides real-time insights that help organizations respond to risks as they develop, rather than waiting for issues to escalate.
- Predictive Modeling: Through statistical models and algorithms, organizations can predict potential risks based on historical trends and behaviors, allowing for timely interventions.
How Machine Learning Transforms Risk Assessment Processes
Machine learning is revolutionizing how organizations approach risk assessment. This subset of artificial intelligence enables systems to learn from previous data sets and refine their predictive capabilities over time. Machine learning algorithms analyze vast data pools to identify patterns, trends, and correlations, enhancing the accuracy of risk assessments. Here are key benefits:
- Automation: By automating data analysis, organizations can significantly reduce the time and manpower required for risk assessments.
- Continuous Learning: Machine learning systems improve continually as they gather more data, helping organizations stay ahead of emerging risks.
- Increased Precision: By utilizing advanced analytical techniques, firms can achieve a higher degree of accuracy in predicting risk probabilities.
The Impact of Big Data on Risk Prediction and Mitigation
The advent of big data has dramatically affected how organizations approach risk prediction and mitigation. Collecting and analyzing large volumes of structured and unstructured data allows businesses to unearth hidden risk factors and respond more effectively. The impact of big data in this context can be summarized as follows:
- Holistic Risk Assessment: Big data integrates various data points, providing a comprehensive view of risk exposure and allowing for more informed decisions.
- Contextual Understanding: By analyzing big data, organizations can understand the external factors contributing to risks, including market dynamics and regulatory changes.
- Customized Risk Mitigation Strategies: With detailed insights available, organizations can tailor their risk mitigation strategies based on unique circumstances rather than a one-size-fits-all approach.
Key Stages of Risk Management Enhanced by Analytics
The risk management process comprises several critical stages, each of which can benefit from the incorporation of analytics:
1. Risk Identification
Risk identification is the first step in the risk management lifecycle. Analytics assists in unearthing risks that may not be immediately apparent, including those that stem from operational inefficiencies, supply chain vulnerabilities, or changes in regulations.
2. Risk Assessment
During the risk assessment phase, analytics tools help quantify the potential impact of identified risks. By leveraging historical data and risk scenarios, organizations can prioritize risks based on their likelihood and impact.
3. Risk Mitigation
Analytics informs risk mitigation strategies by highlighting effective measures based on past data. Businesses can choose the most appropriate response techniques, whether those involve financial planning, process changes, or technology upgrades.
4. Risk Monitoring
Continuous risk monitoring is essential to ensure that risk management strategies remain effective. Analytics provides organizations with the ability to track risk indicators in real-time, enabling quick adjustments as needed.
Leveraging AI for Proactive Risk Monitoring
Artificial Intelligence (AI) plays an instrumental role in promoting proactive risk monitoring. By implementing AI-driven solutions, organizations can enhance their ability to detect threats early and take decisive action. Key advantages include:
- Automated Alerts: AI systems can monitor risk indicators continuously and send alerts for potential issues, significantly reducing human error.
- Predictive Analysis: Advanced analytical models can forecast potential risks, allowing for proactive measures.
- Data Pattern Recognition: AI can quickly identify unusual patterns and behaviors, revealing areas where risks may arise.
Integrating Risk Libraries for Comprehensive Risk Analysis
The creation and maintenance of risk libraries are vital in aligning risk management practices with analytics. Risk libraries serve as centralized repositories of identified risks that organizations can draw upon to inform their analytics strategies. The integration offers multiple benefits:
- Standardization: Risk libraries help standardize risk descriptions, assessments, and mitigation strategies across the organization.
- Facilitated Learning: These libraries allow knowledge sharing for previously encountered risks, enhancing overall risk management practices.
- Data Enrichment: Integrating risk libraries with analytics tools enriches databases, leading to more informed risk assessments.
The Benefits of Structured Risk Analytics Solutions
Structured risk analytics solutions offer organizations the framework to optimize their risk management processes efficiently. Key benefits include:
- Improved Data Quality: Structured solutions ensure data integrity and reduce inconsistencies, which is crucial for accurate risk assessments.
- Enhanced Collaboration: These solutions facilitate better communication among departments, aligning risk management strategies with organizational goals.
- Streamlined Reporting: Organizations can generate detailed reports that provide insights into risk profiles and decision-making efficacy.
Aligning Risk Management Strategies with Business Objectives
Integrating risk management and analytics allows organizations to align their risk management strategies with broader business objectives. This alignment ensures that risk considerations are part of strategic planning, facilitating a holistic approach to risk management. Here are ways to achieve this alignment:
- Stakeholder Engagement: Involving key stakeholders in the risk management process ensures alignment with business priorities.
- Strategic Risk Mapping: Businesses can use analytics to map risks against strategic goals, identifying gaps and opportunities.
- Performance Metrics: Organizations can establish analytics-driven performance metrics that track both risk and business performance.
Improved Communication through Analytics in Risk Management
Effective communication is essential for successful risk management. Analytics enhances this communication by providing clear, data-driven insights that stakeholders can understand. Approaches to improve communication include:
- Visual Analytics: Utilizing visual tools to present risk data clearly helps stakeholders grasp complex information quickly.
- Real-Time Information Sharing: Analytics allows for the dissemination of real-time risk data among teams, ensuring everyone is informed.
- Reporting Dashboards: Creating comprehensive dashboards enhances transparency and facilitates more informed discussions about risk management strategies.
Real-World Examples of Successful Risk Management Analytics
Numerous organizations have successfully harnessed risk management analytics to improve their risk posture. For instance:
- Financial Sector: Many banks utilize predictive analytics to detect fraudulent transactions in real-time, reducing financial losses significantly.
- Manufacturing Industry: Companies implement analytic tools to monitor equipment health and predict failures, minimizing downtime and maintenance costs.
- Retail Business: Certain retailers use analytics to assess supply chain risks and make informed sourcing decisions, optimizing inventory levels.
Overcoming Challenges in Implementing Risk Analytics
While the benefits of analytics in risk management are significant, organizations must address several challenges during implementation, such as:
- Data Silos: Integrating data across departments can be difficult, as many organizations have siloed systems unable to share data seamlessly.
- Skill Gaps: Adequate training is required for employees to leverage analytics tools effectively and interpret complex data accurately.
- Cultural Resistance: Organizations may face resistance to adopting new processes; fostering a culture of data-driven decision-making is crucial.
Future Trends in Analytics for Risk Management Decision-Making
The future of risk management and analytics is set to evolve rapidly as technology continues to advance. Key trends to watch include:
- Integration of Blockchain: This technology can enhance data integrity, enabling more secure risk management processes.
- Augmented Analytics: Combining AI and machine learning will allow more sophisticated analytical capabilities, streamlining decision-making processes.
- Focus on Cybersecurity: As digital threats grow, organizations are increasingly incorporating analytics to bolster their cybersecurity postures.
- Cross-Industry Collaboration: Companies will increasingly share insights and strategies across sectors, promoting comprehensive risk understanding.
To conclude about - analytics in risk management - risk management and analytics
As we have explored, the intersection of analytics in risk management significantly improves decision-making processes within organizations. Embracing data-driven insights equips businesses with the tools needed to proactively identify, assess, and mitigate risks. From leveraging machine learning algorithms to analyzing big data sets, organizations can enhance their risk management frameworks substantially.
In today’s complex business environment, the necessity of integrating robust analytics into risk management systems cannot be overstated. Companies that invest in structured analytics solutions position themselves to navigate uncertainties effectively, ensuring long-term sustainability and growth. Continued evolution in technology and data analytics promises even greater advancements in risk management practices. As such, prioritizing the development and implementation of analytics in risk management will remain a cornerstone of successful business strategy.
Si quieres conocer otros artículos parecidos a How can analytics enhance risk management decision-making puedes visitar la categoría Management Productivity.
Leave a Reply