Population and Sampling: Your Complete Guide to Research
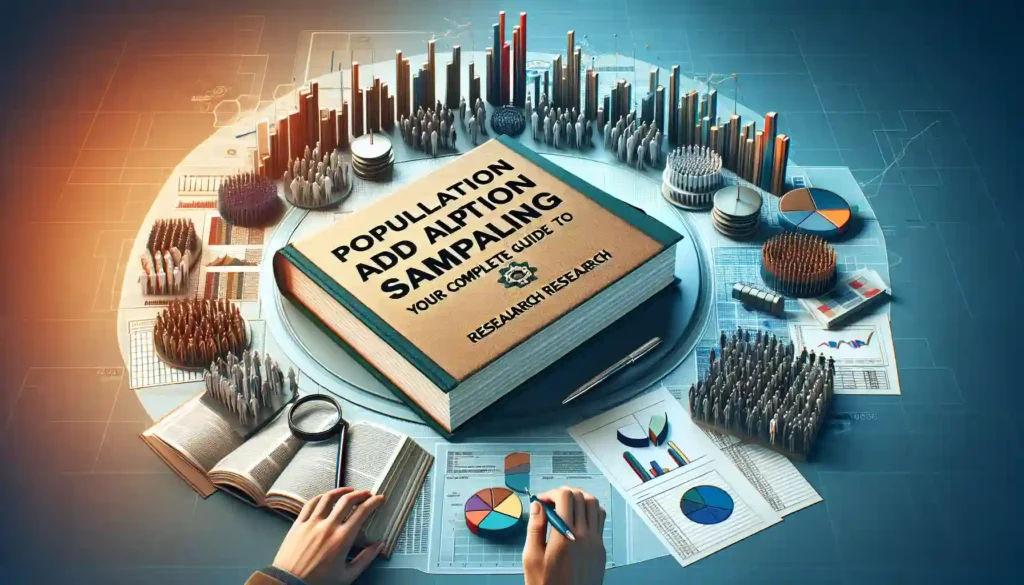
The concept of population and sampling is fundamental in the realm of market research. Researchers need to define their population clearly, as it serves as the foundation for gathering data and drawing conclusions. This population may not solely consist of human beings; it could also include objects, organizations, animals, or even chemical compounds, all sharing specific characteristics relevant to the study. Understanding the definition of the population is necessary for effective research, ensuring that all variables are accounted for and analyzed correctly.
As researchers embark on their studies, the method of data collection from populations significantly impacts the results. In scenarios where the population is relatively small or easily accessible, obtaining data directly from the entire group is often feasible. However, when faced with larger populations, sampling becomes essential. This allows researchers to gather valuable insights from smaller, manageable subsets that reflect the overall population, making the research not only practical but also manageable. In the following sections, we will dive deeper into the intricacies of population and sampling, exploring their definitions, methods, and applications in various fields.
- Understanding Population in Market Research: Beyond Just People
- The Importance of Data Collection from Populations
- Types of Sampling Methods: An Overview
- The Role of Sample Size: Why It Matters in Research
- Representativeness in Sampling: Guaranteeing Accuracy
- Common Pitfalls in Sampling: Avoiding Bias and Inaccuracy
- Practical Applications of Population and Sampling in Different Fields
- Tips for Effective Population and Sampling Strategies in Research
Understanding Population in Market Research: Beyond Just People
The term population in market research goes beyond merely indicating a group of individuals. It embodies all elements pertinent to the study at hand. This broad definition ensures researchers encompass all relevant data points for a comprehensive understanding. In a market research setting, a population could be defined as consumers, businesses, or even specific demographic segments that share common attributes. The demographic characteristics might include age, location, income level, and buying behavior, among others. Recognizing the full scope of a population allows researchers to refine their questioning and enhance data collection processes.
When considering the population and sampling approach, it is crucial to differentiate between the target population and the accessible population. The target population encompasses everyone the researchers aim to study, while the accessible population includes those members available for sampling. Defining a clear target population helps researchers establish parameters for selecting samples, ensuring they obtain data that is indicative of the broader group. Through this understanding, researchers can pinpoint the appropriate strategies for data collection, whether they require exhaustive analysis or can pivot to sampling methods.
The Importance of Data Collection from Populations
Data collection from a population delivers numerous advantages, predominantly the potential for heightened accuracy in research findings. When data is gathered from every member of a population, it fosters a more reliable sense of understanding about behavior patterns, opinions, and preferences. Collecting data directly allows researchers to minimize bias, as they can ensure that every subgroup within the population is accounted for and that diverse perspectives are included in the analysis.
Additionally, gathering data from the entire population provides an in-depth view of the variables at play. Such thorough insights empower researchers to identify correlations and trends that would otherwise remain hidden in smaller subsets. However, complete data collection may prove impractical due to time, cost, and resource constraints. Thus, while comprehensive data gathering is ideal, understanding when to pivot to sampling techniques remains essential.
When to Use Sampling in Research: A Necessary Shift
Sampling becomes necessary when the population under scrutiny is too vast or unwieldy for practical data collection. In cases where time and resource limitations exist, researchers must engage in sampling as a strategic approach to gather insights efficiently. Instead of attempting to penetrate the entire population, which may be costly and time-consuming, researchers can focus on a smaller subset that accurately reflects the characteristics of the more extensive group.
Moreover, sampling can expedite the research process without compromising the validity of the conclusions drawn. With well-calibrated sampling techniques, one can obtain results that are not only representative but also statistically significant. As a result, understanding when to transition from full population data collection to sampling protocols is paramount in crafting an efficient research methodology.
Types of Sampling Methods: An Overview
Two principal categories of sampling methods exist: probability sampling and non-probability sampling. Each type has its merits, and the choice between them will often depend on the research goals, the nature of the population, and the resources available.
Probability Sampling Techniques: Ensuring Randomness
Probability sampling is characterized by the random selection of participants, providing every member of the population an equal opportunity to be included in the sample. This approach aims to minimize bias and enhances the generalizability of findings. Below are some common probability sampling techniques:
- Simple Random Sampling: Every member has an equal chance of selection, typically achieved through random number generators or draw methods.
- Systematic Sampling: Researchers select every nth member from a randomly ordered list of the population.
- Stratified Sampling: The population is divided into distinct subgroups, and random samples are taken from each subgroup. This method ensures all segments are proportionately represented.
- Cluster Sampling: Entire clusters or groups within the larger population are randomly selected instead of individuals, which can be practical in geographically dispersed populations.
Exploring Non-Probability Sampling Methods: When Randomness Isn’t Key
While probability sampling is advantageous for achieving random selection, non-probability sampling methods can also be valuable in certain research scenarios. These methods do not guarantee each member of the population has an equal chance of selection, leading to potential bias but often lower costs and operational simplicity. Common non-probability sampling techniques include:
- Convenience Sampling: Participants are selected based on ease of access. This method is quick and cost-effective but can lead to biased findings.
- Quota Sampling: Researchers ensure certain characteristics are represented by selecting a predefined number of participants from each subgroup.
- Purposive Sampling: Participants are chosen based on specific criteria relevant to the study, aiming for in-depth understanding, rather than breadth.
- Snowball Sampling: Existing study participants assist in recruiting new subjects, which is commonly utilized in populations that are difficult to access or hidden.
The Role of Sample Size: Why It Matters in Research
One of the most critical components of both population and sampling is determining the appropriate sample size. The sample size profoundly influences the reliability and validity of research findings. A larger sample size increases the chances of obtaining a more accurate representation of the population. It also minimizes the margin of error, enhancing statistical power and allowing for more significant confidence in results.
On the other hand, a sample that is too small can result in unreliable conclusions that lack statistical significance. It raises the risk of sampling bias, where the selected sample does not adequately reflect the population. Consequently, as researchers navigate their studies, they must diligently consider their sample size to ensure sufficient data yet remain within practical constraints regarding time and resources.
How to Calculate Sample Size: Tools and Techniques
Calculating the optimal sample size can be achieved using various formulas and statistical tools. The formula typically incorporates factors such as the estimated proportion of the population, the desired confidence level, and the acceptable margin of error. Here are some commonly utilized approaches for sample size calculation:
- Using Standard Formulas: Researchers often use the formula n = (Z^2 * p * (1-p)) / e^2, where n is the sample size, Z is the Z-score corresponding to the desired confidence level, p is the estimated proportion of success, and e is the margin of error.
- Software and Online Calculators: Many statistical packages and online calculators can facilitate accurate calculations, streamlining the process for researchers.
- Referencing Sample Size Tables: Certain resources provide pre-calculated sample sizes based on formulaic approaches, allowing researchers to find appropriate sizes according to population characteristics.
Representativeness in Sampling: Guaranteeing Accuracy
One of the primary goals of employing effective sampling techniques is to achieve representativeness. A sample that accurately reflects the diverse characteristics of the population can provide insights that are valid and applicable across the broader scope of the study. The concept of representativeness hinges on the alignment of the sample’s demographic, social, and behavioral attributes with those of the population it aims to reflect.
To enhance representativeness, researchers can employ stratified sampling methods and ensure that all relevant subgroups are adequately represented within the sample. By doing so, researchers help minimize biases and improve the generalizability of their findings, further solidifying the relationship between sampling strategies and accurate representation.
Common Pitfalls in Sampling: Avoiding Bias and Inaccuracy
Sampling, while an essential strategy in research, poses risks and challenges. Several common pitfalls must be recognized and avoided:
- Selection Bias: This occurs when certain members of the population have a higher chance of being selected than others, skewing results. Using random sampling methods can help mitigate this risk.
- Non-Response Bias: When individuals selected for the sample do not respond, it may lead to distorted results. Researchers should implement strategies to encourage responses, such as follow-up reminders or incentives.
- Overgeneralization: Extrapolating findings from a small sample to the entire population can lead to misleading conclusions. Researchers must clearly outline the limitations of their findings and remain within the context of their study group.
- Sample Size Confusion: Understanding the balance between sample size and representativity is crucial. A large sample does not automatically ensure accuracy; researchers must also consider the diversity of the sample to ensure it reflects the population.
Practical Applications of Population and Sampling in Different Fields
The practical applications of population and sampling extend across various fields, showcasing the versatility of these concepts. Market research, healthcare, social sciences, and environmental studies all benefit from effective population and sampling strategies. For instance:
- Market Research: Understanding consumer behavior is crucial in the marketplace. Companies rely on sampling to gauge preferences and perceptions, applying insights to product development and marketing strategies.
- Healthcare Studies: Researchers conduct studies on diseases and treatment responses, utilizing samples to infer trends and correlations in larger populations, ultimately improving patient care.
- Social Science Research: Academics often rely on sampled populations to investigate social behaviors, attitudes, and trends, generating findings that contribute to theory development and policy-making.
- Environmental Studies: When analyzing ecological impacts, sampling allows researchers to assess biodiversity and environmental health within specific ecosystems without needing to sample every organism.
Tips for Effective Population and Sampling Strategies in Research
Successfully navigating the landscape of population and sampling requires meticulous planning and execution. Here are some practical tips to enhance the research process:
- Define Clear Objectives: Establish what you aim to achieve through your research. Specific goals will help guide your population definition and sampling methods.
- Employ Mixed Methods: In some instances, a combination of qualitative and quantitative approaches can yield richer insights, capturing a diverse set of data points.
- Pilot Testing: Consider conducting pilot tests on your sampling methods before the full rollout. This will allow you to refine your approach, enhance reliability, and identify potential issues.
- Continually Review and Adjust: As the research progresses, remain open to reassessing your sampling strategy based on preliminary results and feedback.
To conclude about - population and sampling
Mastering the principles of population and sampling is essential for conducting effective and reliable research. Understanding the broader implications of population definition, the importance of effective sampling strategies, and the risks associated with various sampling methods are crucial for researchers across multiple fields. By implementing thoughtful sampling techniques, determining appropriate sample sizes, and striving for representative samples, researchers can draw meaningful conclusions that resonate within comprehensive studies. Ultimately, adept use of these concepts will empower researchers to navigate the data landscape more effectively, producing insights that inform better practices, policies, and understanding.
Si quieres conocer otros artículos parecidos a Population and Sampling: Your Complete Guide to Research puedes visitar la categoría Surveys Sampling Methods.
Leave a Reply