Stratified Random Sampling: Definition, Examples, and Guide
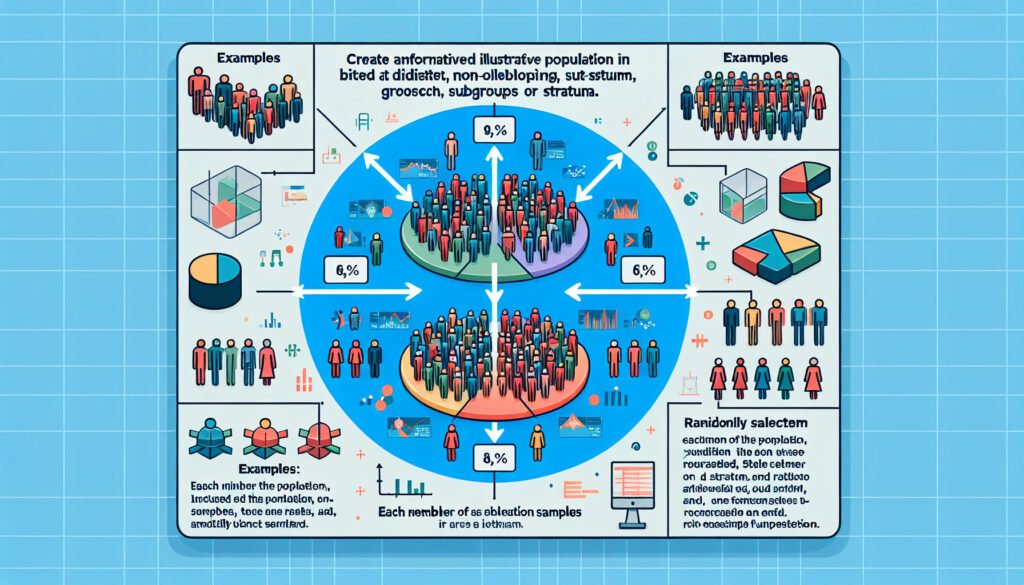
Stratified random sampling is an essential technique in the realm of research and data collection that focuses on achieving precise and representative samples from diverse populations. By dividing a population into smaller, homogeneous groups known as strata, researchers can ensure that each subgroup is adequately represented, leading to more reliable data. This method is particularly effective in situations where variations amongst the groups can significantly influence the results, thereby showcasing its strength in minimizing bias and enhancing the validity of findings. By utilizing stratification, researchers can make meaningful comparisons between different segments of the population, which can unveil insights that would otherwise remain hidden.
Implementing stratified random sampling requires a thoughtful approach to classification, random selection, and data analysis. While it offers substantial advantages in terms of data quality and representativeness, it can also pose challenges, such as increased complexity and the need for careful management of sample sizes within each stratum. Understanding the nuances of stratification sampling is crucial for researchers looking to leverage this powerful tool effectively. It invites deeper engagement with the material and a broader perspective on collective behaviors and trends, which are vital for data-driven decision-making across various fields.
-
Understanding Stratified Random Sampling: A Comprehensive Overview
- What is Stratified Random Sampling?
- Key Characteristics of Stratified Sampling
- The Importance of Stratification in Research
- How Stratified Random Sampling Works
- Examples of Stratified Random Sampling in Practice
- The Benefits of Using Stratified Random Sampling
- Challenges and Limitations of Stratified Random Sampling
- Comparing Stratified Random Sampling with Other Methods
- A Step-by-Step Guide to Implementing Stratified Random Sampling
- Tips for Maintaining Representation in Stratified Sampling
- Analyzing Results from Stratified Random Samples
- Best Practices for Researchers Using Stratified Random Sampling
Understanding Stratified Random Sampling: A Comprehensive Overview
Stratified random sampling is a method of sampling that aims to ensure that various segments of a population are represented adequately in a study. Unlike simple random sampling, which treats every individual in a population as equally likely to be chosen, stratified sampling divides the population into distinct subgroups (or strata) based on shared attributes such as age, gender, income level, education, or other demographic features. This division into strata allows researchers to capture the diversity of the population and ensures that each subgroup is properly represented in the sample.
The main purpose of stratified sampling is to improve the precision of the estimates derived from the sample, especially when there is a significant difference between strata. By sampling each subgroup independently, researchers can reduce the overall variance of the sample and obtain more reliable and valid results. This method is advantageous in research fields such as social sciences, health studies, market research, and public opinion polling, where understanding the nuances among different groups within a population can lead to more nuanced insights.
What is Stratified Random Sampling?
Stratified random sampling can be defined as a probability sampling technique that aims to improve the accuracy and reliability of sample estimates through the deliberate selection of participants from predefined groups within a population. Each stratum within the population should be internally homogeneous, meaning that the members of each group share similar characteristics, yet they should be heterogeneous when compared to other strata. The stratification process facilitates targeted sampling that reflects the broader population’s diversity while minimizing bias associated with random selection.
This method is typically implemented in two main stages: first, identifying the strata based on relevant characteristics, and second, conducting random sampling within each of these strata. Random selection can occur at various levels, including simple random sampling or systematic sampling methods. The outcome is a sample that mirrors the population's composition, making it easier to analyze trends and draw conclusions about particular subgroups.
Key Characteristics of Stratified Sampling
Understanding the key characteristics of stratified sampling is essential for researchers who want to effectively implement this technique. Some of the most important characteristics include:
- Homogeneity within strata: Members of each stratum should be similar in terms of the characteristic used for stratification, which ensures a clear focus for comparison.
- Heterogeneity between strata: Strata should differ significantly, allowing for in-depth analysis and insights about various groups.
- Random selection: Participants are chosen randomly from each stratum, maintaining the randomness and reducing selection bias.
- Proportional allocation: The size of the sample from each stratum can be proportional to the stratum's size in the population to ensure an accurate representation.
The Importance of Stratification in Research
Stratification is vital in research for several reasons. Firstly, it enhances the reliability of study results by ensuring that both small and large subgroups are adequately represented. This means that researchers can generate more accurate estimates for each subgroup, which is particularly critical when specific subgroups are of primary interest in the study.
Secondly, stratification allows for the examination of subgroup differences, leading to richer and more detailed analyses. With stratified random sampling, researchers can investigate variations in attitudes, behaviors, and outcomes across different strata, offering valuable insights into complex social phenomena or market behaviors.
Lastly, stratification contributes to a more ethical approach to research by guaranteeing that vulnerable or marginalized groups are not overlooked or underrepresented. By consciously planning for representation based on key characteristics, stratified sampling promotes inclusivity and equity in research practices.
How Stratified Random Sampling Works
The process of conducting stratified random sampling involves several structured steps. Key stages include:
- Define the population: Identify the entire group from which you want to draw your sample.
- Identify strata: Segment the population into relevant groups based on shared characteristics.
- Determine the sample size: Decide how large your sample will be and how many participants you want from each stratum.
- Select the participants: Use a random selection method to choose participants from each of the identified strata.
- Collect data: Implement the research methodology to gather data from your selected sample.
This process, when executed thoughtfully, ensures that each stratum is adequately represented, which is critical for achieving the study's goals and generating valid findings.
Examples of Stratified Random Sampling in Practice
To illustrate stratified random sampling examples, consider the following scenarios:
- Educational Research: A researcher studying the effectiveness of a new teaching method might stratify students by grade level (e.g., 1st, 2nd, and 3rd grades) to ensure feedback comes from an appropriate variety of educational experiences.
- Market Research: A company looking to understand consumer preferences may stratify respondents based on demographics such as age, income, and geography to gather a comprehensive picture of market trends.
- Health Studies: In a health-related study, researchers might divide participants into strata based on pre-existing conditions (e.g., diabetic, hypertensive) to assess how different treatment methods impact various conditions.
The Benefits of Using Stratified Random Sampling
The advantages of stratified random sampling are numerous, particularly in enhancing the robustness of research findings. Some key benefits include:
- Improved accuracy: The method ensures that samples closely represent the population, leading to more accurate results.
- Reduced variance: By accounting for differences across strata, researchers can lower the variability of estimates, improving the precision of findings.
- Focused analysis: Stratification allows researchers to analyze and compare results between different groups, providing deeper insights.
- Cost-effective: While the initial design may involve more complexity, overall research costs can be reduced by improving the validity of findings, thus minimizing potentially expensive errors in data interpretation.
Challenges and Limitations of Stratified Random Sampling
Despite its many benefits, stratified sampling does come with challenges and limitations. Researchers must be aware of the following issues:
- Complexity: Designing and implementing stratified sampling can be more complicated than other sampling methods, requiring careful planning and additional resources.
- Difficulty in identifying strata: Accurately defining strata can be challenging, especially if the classifications are not clear-cut or if the population is diverse.
- Sample size concerns: If a stratum has a very small population, achieving a statistically significant sample size may be difficult.
- Bias risk: Insufficient randomization within strata can introduce bias, undermining the benefits of stratification.
Comparing Stratified Random Sampling with Other Methods
To understand the unique value of stratified random sampling, it’s useful to compare it with other sampling methods, such as simple random sampling and cluster sampling. Here’s a breakdown of how these methods differ:
Method | Overview | Advantages | Disadvantages |
---|---|---|---|
Simple Random Sampling | Every individual in the population has an equal chance of being selected. | Easy to implement; reduces bias. | May not represent all subgroups adequately. |
Cluster Sampling | The population is divided into clusters, and entire clusters are randomly selected. | Cost-effective for large populations. | Higher variance; less representation of diverse groups. |
Stratified Random Sampling | Population divided into strata and random samples taken from each. | Accurate representation of subgroups; reduces bias. | More complex to administer; requires careful stratum definition. |
A Step-by-Step Guide to Implementing Stratified Random Sampling
For researchers eager to apply stratified random sampling effectively, adhering to a structured process is critical. Here’s a guide to streamline implementation:
- Define your research goals: Determine what you aim to achieve with your study, guiding your approach to stratification.
- Identify the population: Clearly delineate the population you wish to study.
- Determine stratification criteria: Decide on the characteristics that will define the strata (e.g., age, income, education level).
- Decide sample size: Calculate how many participants you need to ensure statistical significance.
- Allocate sample sizes to strata: Choose whether to use proportionate or disproportionate allocation based on research needs.
- Randomly select participants: Employ a random selection technique for choosing subjects from each stratum.
- Gather and analyze data: Perform data collection and analysis, disaggregating results by strata where necessary.
Tips for Maintaining Representation in Stratified Sampling
To effectively maintain representation in stratified sampling, researchers should consider the following tips:
- Consistency in strata: Ensure that the strata remain consistent and meaningful through the research process.
- Regular updates: Periodically review the population and strata to adapt to any changes that may occur over time.
- Thorough documentation: Document the criteria and processes used for stratification and selection to ensure reproducibility and transparency.
- Engage stakeholders: Involving community members or those affected by the research can help identify the most relevant strata.
Analyzing Results from Stratified Random Samples
Analyzing results obtained through stratified random sampling entails several crucial considerations. Researchers should:
- Segment data: When analyzing results, segment data by strata to identify patterns or differences among groups.
- Adjust for weights: If using disproportionate sampling, adjust analyses to account for the weights of different strata.
- Report findings clearly: Ensure that analyses are presented transparently, emphasizing differences across strata as well as overall insights.
Best Practices for Researchers Using Stratified Random Sampling
Finally, researchers employing stratified random sampling should adhere to best practices, including:
- Pre-testing: Conduct a pilot test of the sampling methodology to identify potential issues before the full-scale study.
- Training for data collectors: Ensure that individuals collecting data understand the importance of maintaining stratification during the data collection process.
- Continual assessment: Evaluate and adjust strategies throughout the study to adapt to any unforeseen challenges with representation.
To conclude about - stratification sampling
In summary, stratification sampling is a sophisticated and valuable technique that enhances the reliability and validity of research findings. By focusing on detailed subgroup sampling, researchers can gain insights into population dynamics that would be obscured in less structured sampling methods. With the right approach and adherence to best practices, employing a stratified survey example can yield rich, actionable data.
Through stratified examples, we have seen how this method can be applied in various fields, confirming its adaptability and effectiveness. The example of stratified random sampling showcases how intentional selection can yield substantial benefits in understanding complex issues, whether in educational research, market analysis, or health studies.
By exploring a stratified random example, researchers not only navigate the intricacies of stratification in sampling but also highlight the importance of tailored research methodologies. Striving for a well-rounded understanding and implementation of stratified random sampling example methods further solidifies the validity of research outcomes, effectively paving the way for informed decisions and findings.
Ultimately, the journey from theoretical understanding to practical application of stratified random sampling underscores its significant role in advancing research integrity and population representation.
Si quieres conocer otros artículos parecidos a Stratified Random Sampling: Definition, Examples, and Guide puedes visitar la categoría Surveys Sampling Methods.
Leave a Reply