Surveys and Statistics: Analyzing Survey Data Methods
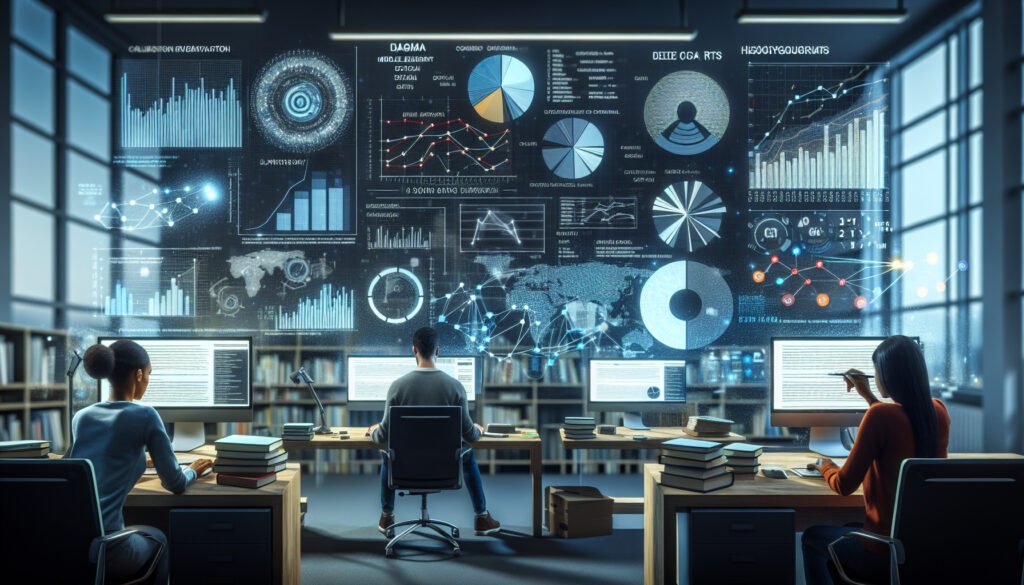
Surveys and statistics play a crucial role in understanding consumer behavior, preferences, and market dynamics. With the surge of digital data collection methods, organizations have more access than ever to extensive survey statistics. Yet, the challenge lies not just in gathering this data, but in effectively transforming it into actionable insights through various data analysis techniques for surveys.
Utilizing the right methods for analyzing survey data can significantly influence the decision-making processes within a business. The intricate relationship between collected data and meaningful analysis shapes how companies approach market research and customer engagement, making it essential to grasp these concepts thoroughly.
- Understanding Surveys and Statistics: Key Concepts and Importance
- The Role of Statistical Analysis in Survey Data
- Transforming Raw Data into Meaningful Insights
- Essential Steps in Analyzing Survey Data
- Importance of Sampling in Survey Statistics
- Defining Null and Alternative Hypotheses in Research
- Utilizing Benchmarks for Standardized Results
- Common Statistical Methods for Analyzing Survey Data
- Exploring Regression Analysis: Uncovering Relationships
- Understanding T-Tests: Comparing Averages
- The Power of ANOVA: Analyzing Variance Among Groups
- Cluster Analysis: Grouping and Segmenting Respondents
- Advanced Statistical Techniques: Factor and Conjoint Analysis
- Interpreting Results and Drawing Actionable Insights
- Tools for Simplifying Statistical Analysis in Surveys
- Enhancing Analysis with Qualtrics Stats iQ and Other Tools
- Best Practices for Presenting Survey Data Findings
- Challenges in Analyzing Survey Data and How to Overcome Them
Understanding Surveys and Statistics: Key Concepts and Importance
To effectively grasp the relationship between surveys and statistics, it’s essential to understand the fundamentals of both fields. Surveys are structured methods of data collection that can yield quantitative or qualitative insights through questionnaires, interviews, or polls. The role of statistics in this context is to systematically analyze the collected data to identify patterns, trends, and relationships among variables. This analysis not only aids in validating assumptions but also enhances the capability of organizations to respond to the needs of their target audiences.
The importance of effectively analyzing survey data cannot be overstated. Businesses, researchers, and policymakers rely on statistical analyses to drive their initiatives forward based on factual data. Conducting appropriate statistical analyses ensures that findings are statistically significant and generalizable across a broader population, thus instilling confidence in the validity of the results.
The Role of Statistical Analysis in Survey Data
Statistical analysis serves as the backbone for extracting insights from survey data. It allows researchers to make sense of complex data sets, ensuring that the conclusions drawn are reliable and actionable.
- Data Validation: Statistical analysis helps in validating the data collected, ensuring it accurately reflects the population being studied.
- Identifying Trends: It assists in recognizing trends and patterns over time, which can inform future business strategies.
- Decision Making: Statistical outcomes inform critical decisions, from product development to marketing strategies.
Ultimately, the role of statistical analysis in survey data is not just to compute numbers but to transform those numbers into a narrative that can guide and influence decisions.
Transforming Raw Data into Meaningful Insights
The transition from raw data to meaningful insights involves a systematic approach. Once data is collected, it requires cleaning, which entails correcting errors, removing missing or irrelevant information, and ensuring that it is in a suitable format for analysis. Following this, the next phase is data exploration, where initial analyses are performed to grasp basic patterns and establish hypotheses.
Utilizing descriptive statistics helps summarize the data, providing a clearer picture of the trends in the dataset. Visualization tools (graphs, charts) can also aid in comprehensively presenting findings during this stage. After establishing the foundation, more complex analyses are employed to examine relationships between variables.
Essential Steps in Analyzing Survey Data
Analyzing survey data involves a series of essential steps designed to ensure accurate and reliable results. These steps include:
- Define Objectives: Clearly outline what you want to learn from the survey data.
- Data Collection: Utilize well-structured surveys that effectively gather the needed information.
- Data Cleaning: Remove inaccuracies and irrelevant data from your dataset to ensure reliability.
- Exploratory Data Analysis (EDA): Begin by examining the data's basic features to identify patterns.
- Data Analysis: Employ appropriate statistical methods to derive insights.
- Interpret Results: Draw actionable insights from data without jumping to conclusions.
- Present Findings: Share results in a clear and compelling manner to the stakeholders.
Each of these steps is crucial in ensuring the integrity and usability of the data, recovering the benefits of thorough analysis.
Importance of Sampling in Survey Statistics
A critical aspect of survey statistics involves understanding the role of sampling. Sampling refers to selecting a representative portion of the population to participate in the survey, which ensures that the data collected reflects the views and experiences of the entire group. Accurate sampling is key to generalizing results and avoiding biases that may mislead interpretations.
Factors to consider in sampling include:
- Sample Size: A larger sample size generally yields more reliable results.
- Sampling Method: Methods can include random sampling, stratified sampling, or convenience sampling, each with its advantages.
- Diversity: Ensure the sample population reflects the varied characteristics of the larger population.
By carefully defining the sampling strategy, researchers can significantly enhance the validity of their survey results.
Defining Null and Alternative Hypotheses in Research
Another pivotal aspect of analyzing survey data is formulating null and alternative hypotheses. The null hypothesis (H0) posits that there is no effect or relationship between the variables being studied, while the alternative hypothesis (H1) proposes that a significant effect or relationship does exist. Establishing these hypotheses is critical in guiding the analysis and determining the direction of the research.
The process involves:
- Formulating Hypotheses: Clearly state both the null and alternative hypotheses based on the objectives of the analysis.
- Choosing the Right Test: Select the appropriate statistical test based on the type of data and hypothesized relationship.
- Evaluating Results: Use statistical methods to evaluate whether to accept or reject the null hypothesis based on the evidence from the data.
Through this structured approach, researchers can derive significant conclusions that are essential for evidence-based decision-making.
Utilizing Benchmarks for Standardized Results
Benchmarking involves comparing survey results against a standard or external metric, enabling researchers to contextualize findings. This comparison aids organizations in determining their performance relative to industry standards or similar groups, thus illuminating areas needing improvement.
Key advantages of benchmarking include:
- Establishing Standards: Helps in setting performance standards for future surveys.
- Identifying Trends: Enables detection of trends that align with or deviate from the norm.
- Informing Strategic Decisions: Provides a context for analyzing survey results, informing strategic planning.
This method highlights variances that could indicate opportunities for growth or need for intervention.
Common Statistical Methods for Analyzing Survey Data
Several methods for analyzing survey data exist, each serving specific purposes. Common techniques include:
Exploratory Data Analysis (EDA)
EDA focuses on summarizing main characteristics and visualizing data. It helps in understanding behaviors and detecting patterns without relying on formal statistical modeling.
Descriptive Statistics
Traditionally involves calculating measures like mean, median, mode, and standard deviation. These metrics provide insight into general trends within the data.
Inferential Statistics
Methods such as confidence intervals and hypothesis testing are employed to make predictions or inferences about a population based on sample data.
Regression Analysis
Regression allows for analyzing relationships between variables, helping to make predictions or understand the impact of varying factors.
By employing these methods, researchers can navigate through data complexities and extract valuable insights.
Exploring Regression Analysis: Uncovering Relationships
Regression analysis is a powerful statistical tool used to understand relationships between a dependent variable and one or more independent variables. This method is particularly vital in determining how changes in the predictors influence outcomes.
There are various types of regression analysis, including:
- Linear Regression: Examines the linear relationship between independent and dependent variables.
- Multiple Regression: Assesses the impact of multiple predictors on a single outcome variable.
- Logistic Regression: Suitable for analyzing binary outcomes, determining the probability of a categorical dependent variable.
By employing regression analysis, businesses can uncover meaningful relationships between different factors, leading to informed decision-making.
Understanding T-Tests: Comparing Averages
T-Tests are a statistical method employed to compare the means of two groups to determine if they are statistically different from each other. This is particularly useful in survey data when assessing the impact of different variables on a particular outcome.
Types of T-Tests include:
- Independent T-Test: Comparing means from two unrelated groups.
- Paired T-Test: Comparing means from the same group at different times.
- One-Sample T-Test: Comparing the sample mean against a known value.
This method is crucial for understanding whether differences observed in survey results are significant and warrant further exploration.
The Power of ANOVA: Analyzing Variance Among Groups
Analysis of Variance (ANOVA) is another vital statistical method used to compare the means of three or more groups. It helps determine whether any of the group means are significantly different from one another.
Benefits of using ANOVA include:
- Efficiency: Simultaneously tests multiple groups instead of using multiple T-Tests, reducing Type I error probability.
- Assessing Interaction: Can explore the interaction between different independent variables affecting the dependent variable.
ANOVA provides a framework for understanding the variance among multiple groups, which may arise from survey data.
Cluster Analysis: Grouping and Segmenting Respondents
Cluster analysis is a statistical technique utilized to group respondents based on their characteristics or responses. This method enhances understanding of common patterns amongst a subset of respondents, paving the way for targeted strategies.
Key applications of cluster analysis include:
- Market Segmentation: Identifying distinct customer segments that share similar traits.
- Personalization: Tailoring products or services to meet the specific needs of different customer groups.
By effectively segmenting respondents, organizations can tailor their offerings more closely to their target audience’s preferences.
Advanced Statistical Techniques: Factor and Conjoint Analysis
Advanced statistical techniques provide deeper insights into customer preferences and behaviors. Key methods include:
Factor Analysis
Factor analysis identifies underlying variables (factors) that explain the data patterns. This is especially useful in survey responses spanning multiple questions that can be grouped under broad themes.
Conjoint Analysis
This technique assesses consumer preferences by presenting them with product or service options, allowing researchers to determine which features are most valued by consumers. It helps in optimizing product offerings based on customer desires.
Employing these advanced techniques equips researchers with the ability to unearth nuanced insights that standard analysis may overlook.
Interpreting Results and Drawing Actionable Insights
Once statistical analyses are complete, interpreting the results becomes pivotal. It involves making sense of statistical outputs in terms of their real-world implications. Researchers should focus on:
- Statistical Significance: Assess whether the findings are statistically significant, indicating reliability.
- Practical Significance: Determine the practical implications and expected impact of the findings.
- Recommendations: Formulate actionable recommendations based on the insights gained from the analysis.
Effective interpretation not only provides clarity but also enhances the likelihood of implementing changes based on survey findings.
Tools for Simplifying Statistical Analysis in Surveys
Several tools can simplify statistical analysis, making them more accessible to non-experts. Some of these include:
- Qualtrics Stats iQ: Offers automated analysis and visualization, simplifying complex data interpretations.
- SPSS: A comprehensive program used for statistical analysis that provides extensive capabilities for manipulating and analyzing data.
- R and Python: Open-source programming environments that provide numerous packages tailored for advanced statistical analysis.
Utilizing these tools can lead to more streamlined analysis processes while retaining rigorous methodologies.
Enhancing Analysis with Qualtrics Stats iQ and Other Tools
Qualtrics Stats iQ stands out as a user-friendly solution that empowers users to conduct sophisticated analyses without extensive statistical training. Its key features include:
- Smart Analysis: Automates analysis, providing insights and visualizations based on user selections.
- Customizable Reports: Users can tailor reports to highlight critical findings important for their unique contexts.
- Predictive Intelligence: Offers forecasts and insights based on historical data and trends.
This tool enhances the efficiency of survey data analysis, democratizing access to sophisticated statistical methods.
Best Practices for Presenting Survey Data Findings
When sharing the findings from survey analyses, it's crucial to adhere to best practices that ensure clarity and impact. Key strategies include:
- Visualizations: Employ charts and graphs to present data visually, making it easier for audiences to comprehend the findings.
- Clear Language: Use accessible language, avoiding excessive jargon, to ensure findings are understandable to all stakeholders.
- Narrative: Craft a narrative around the data, discussing implications and recommendations alongside the numbers.
Effective presentation of data not only conveys the findings but also serves to engage stakeholders in the decision-making process.
Challenges in Analyzing Survey Data and How to Overcome Them
Despite the importance of analyzing survey data, various challenges can arise. Common issues include:
- Data Quality: Incomplete or inaccurate data can lead to misleading conclusions. Solution: Implement rigorous data cleaning processes.
- Statistical Misinterpretation: Misunderstanding statistical tests may lead to erroneous insights. Solution: Invest in training or consult statistical experts.
- Generalizability: Results from non-representative samples can mislead. Solution: Ensure robust sampling techniques are utilized.
Being aware of these challenges allows researchers to adopt strategies that mitigate risks and enhance the integrity of their analytical processes.
To conclude about - surveys and statistics
Analyzing survey data through robust data analysis techniques for surveys not only enhances decision-making but also paves the way for innovative solutions in business. The various methods for analyzing survey data contribute to understanding consumer needs and behaviors, vital for any organization seeking to thrive in a competitive landscape. By employing statistical analysis effectively, businesses can transform collected survey data into survey statistics that drive insightful conclusions and foster strategic growth.
Si quieres conocer otros artículos parecidos a Surveys and Statistics: Analyzing Survey Data Methods puedes visitar la categoría Surveys Sampling Methods.
Leave a Reply