Survey Biases: Key Types Every Researcher Should Know
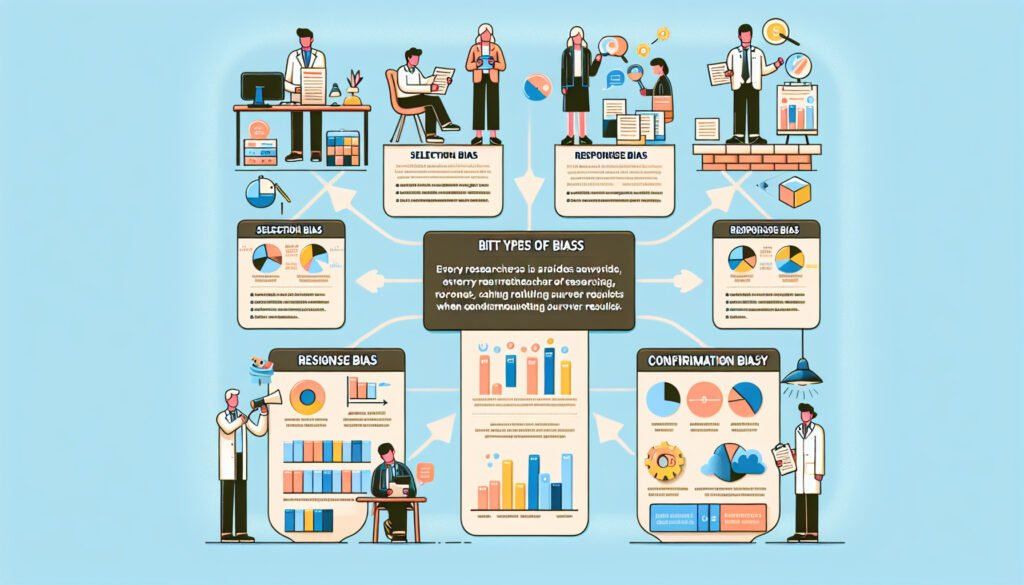
Market research reports and surveys, both online and offline, are vital tools for businesses to understand their audiences at scale, uncovering customer preferences, demographics, and behaviors to tailor products and services accordingly. However, survey bias can significantly distort data interpretation and responses, arising from factors like selection, response, interviewer influence, and the participants' own biases. Understanding the types of biases in surveys is essential for researchers to ensure they collect accurate and meaningful data.
Addressing survey biases is crucial in maintaining the integrity of research findings. In this extensive article, we will explore the various types of survey bias, dissect the implications of these biases on research validity, and discuss strategies for mitigating their effects. By recognizing the nuances in bias within survey methodologies, researchers can improve the reliability of their findings and ultimately make better-informed business decisions.
- Understanding Survey Bias: The Impact on Research Validity
- Selection Bias: How Poor Sampling Affects Results
- Sampling Bias: The Risks of Non-Representative Samples
- Non-Response Bias: Challenges with Participant Engagement
- Response Bias: Distortions in Participant Answers
- Acquiescence Bias: The Tendency to Agree
- Social Desirability Bias: Responding to Social Norms
- Question Wording Bias: The Influence of Survey Language
- Order Effects: How Question Sequence Changes Responses
- Mitigating Survey Bias: Strategies for Researchers
- The Role of Technology in Reducing Bias
- Best Practices for Effective Survey Design
- Case Studies: Real-World Examples of Survey Bias
Understanding Survey Bias: The Impact on Research Validity
Survey bias refers to systematic errors that influence the results of a survey, rendering the outputs unreliable. These biases can lead to misleading conclusions, making it imperative for researchers to be cognizant of the various forms that bias can take. When bias in surveys occurs, it disturbs the balance and accuracy of data, thus undermining the overall validity of research. Each type of bias has unique characteristics and implications, and understanding them can significantly enhance the robustness of research methodologies.
One of the primary concerns with types of biases in surveys is how they skew results. This could manifest as the tendency for certain demographics to be overrepresented or underrepresented, the influence of survey language on responses, or even social influences that shape how participants respond. Recognizing these biases equips researchers with the insight needed to implement effective corrective measures, ensuring their findings resonate accurately with the targeted demographic.
Selection Bias: How Poor Sampling Affects Results
Selection bias occurs when the participants who are selected for a survey do not adequately represent the intended population. This type of bias can arise from a variety of sources, such as self-selection, where individuals choose to participate based on their own characteristics or motivations. As a result, the data collected may not reflect the true sentiments of the broader audience.
- Self-selection bias: Participants choose themselves based on personal interest.
- Exclusion bias: Certain groups are systematically excluded from the sample.
- Geographical bias: Focusing on a specific region may yield non-representative data.
Addressing selection bias requires careful planning of sample selection criteria and the use of stratified sampling techniques to ensure diverse representation across relevant demographics. By reducing selection bias, researchers can gather findings that are more reflective of the entire population.
Sampling Bias: The Risks of Non-Representative Samples
Sampling bias occurs when the sample drawn for the survey doesn't accurately reflect the population from which it was taken. This may arise due to poor sampling methodologies or a lack of diligence in ensuring that various subgroups are adequately represented. Such bias can lead to incorrect or biased results that can negatively influence decision-making.
- Purposive sampling: Intentionally including or excluding certain groups can lead to misleading results.
- Convenience sampling: Drawing samples based solely on ease of access may overlook essential demographics.
- Quota sampling: Setting quotas based on specific characteristics can inadvertently lead to imbalances.
Mitigating sampling bias involves utilizing random sampling techniques where every individual in the population has an equal chance of being selected. This approach enhances the representation of diverse perspectives and minimizes the risks associated with non-representative samples.
Non-Response Bias: Challenges with Participant Engagement
Non-response bias occurs when individuals selected for a survey fail to respond, resulting in a skewed representation of the population. The absence of these voices often leads researchers to overlook critical insights that could significantly shape their findings. Non-response bias can stem from various factors, including participant fatigue, lack of awareness, or technical difficulties.
- Item non-response: Some participants skip certain questions, affecting overall data validity.
- Unit non-response: Entire individuals fail to complete the survey.
- Response fatigue: Lengthy surveys can overwhelm participants, driving them to abandon the process.
To combat non-response bias, researchers should prioritize making surveys more engaging, ensuring questions are concise, clear, and easy to respond to. Offering incentives or reminders to encourage participation can also enhance response rates and reduce bias associated with non-response.
Response Bias: Distortions in Participant Answers
Response bias involves inaccuracies in responses given by participants, either due to misunderstanding questions, misrepresentation, or personal biases. This phenomenon can compromise the authenticity of data and result in skewed data interpretations.
- Acquiescence bias: The tendency for respondents to agree with statements regardless of their true opinions.
- Extreme responding: The inclination to choose the highest or lowest options on a scale regardless of context.
- Social desirability bias: Respondents may answer in a manner that they believe is socially acceptable rather than providing their true thoughts.
Understanding the potential for response bias encourages researchers to develop strategies that elicit more honest responses, such as using neutral wording and avoiding leading questions. Properly crafting questions and establishing a trusting rapport can facilitate more accurate responses and enrich data quality.
Acquiescence Bias: The Tendency to Agree
Acquiescence bias refers to the tendency of survey respondents to agree with statements or questions regardless of their actual stance. This phenomenon can particularly impact surveys where agreement scales are utilized. Participants may default to a positive answer instead of providing their authentic perspective.
Challenges associated with acquiescence bias can lead researchers to overestimate consensus levels or misinterpret the data collected. To minimize this bias, researchers should critically evaluate their survey content and consider including reverse-coded items to encourage varied responses, thereby creating a more balanced dataset.
Social desirability bias occurs when respondents provide answers they perceive to be more socially acceptable rather than their true feelings or experiences. This is especially prevalent in sensitive topics such as health or personal behavior, where respondents may fear judgment or criticism.
To address social desirability bias, researchers can adopt various techniques to foster candidness in responses, such as ensuring anonymity and confidentiality. Additionally, employing indirect questioning techniques can help participants feel more comfortable sharing their genuine opinions.
Question Wording Bias: The Influence of Survey Language
Question wording bias emerges when the phrasing of questions unintentionally leads respondents toward a particular answer. Ambiguous language, loaded terms, or unclear instructions can significantly skew responses, ultimately compromising the data's accuracy.
- Leading questions: Questions that suggest a particular response, thereby influencing how a respondent answers.
- Loaded questions: Questions that contain assumptions or bias, prompting defensive responses.
Recognizing question wording bias is crucial for researchers, as carefully constructed questions can elicit more accurate and representative responses. Rigorous pre-testing of survey questions can identify potential biases and aid in refining the language used.
Order Effects: How Question Sequence Changes Responses
Order effects refer to the potential for respondents to be influenced by the sequence in which questions are presented. The initial questions can prime respondents, affecting their subsequent answers. This bias can distort perspectives and lead to inconsistency in responses.
To reduce the impact of order effects, researchers should randomize question sequences. This strategy helps ensure that no particular order influences the responses gathered, fostering more reliable data.
Mitigating Survey Bias: Strategies for Researchers
Mitigating survey biases requires a multifaceted approach, incorporating careful planning and strategic execution. Here are several practical strategies researchers can employ:
- Develop a clear objective: Defining the purpose of the survey helps align questions with research goals.
- Emphasize randomization: Ensure that sampling methods and question orders are randomized to maintain representation.
- Provide instructions: Clear, concise instructions can reduce respondents’ confusion and lead to more accurate answers.
- Use pilot testing: Conduct trials with a small sample to identify potential biases or issues in survey design prior to major deployment.
- Implement follow-ups: Utilize reminders and follow-ups to enhance participation rates and reduce non-response bias.
The Role of Technology in Reducing Bias
Advancements in technology offer researchers innovative means to mitigate bias in surveys. Online survey platforms provide automated data collection and analytics, allowing for a more streamlined process. These tools can help minimize human error during data collection and enhance the integrity of sample selection.
Additionally, sophisticated algorithms can analyze patterns in responses, identifying potential biases and flags for further review. Leveraging technology ensures that researchers can capture insights in real-time and adapt their methods accordingly to improve the quality of findings.
Best Practices for Effective Survey Design
Implementing best practices in survey design is vital for achieving a robust and trustworthy dataset. Here are essential components to consider:
- Focus on clarity: Use straightforward language and avoid jargon in questions.
- Avoid leading questions: Ensure that questions do not imply a desired response.
- Limit survey length: Keeping surveys concise encourages higher completion rates and engagement.
- Mix question types: Incorporate various types (multiple choice, open-ended) to capture diverse insights.
- Test for bias: Conduct extensive testing for potential biases before launching the survey.
Case Studies: Real-World Examples of Survey Bias
Understanding theoretical concepts of survey bias is essential, but analyzing real-world applications can illustrate the implications of these biases. Here are notable case studies that showcase common biases:
- Health Survey Misrepresentation: A health survey targeted at young adults resulted in a significant sampling bias because it predominantly attracted individuals from higher socioeconomic backgrounds. The findings led to skewed conclusions about public health behaviors, leading policymakers to focus on issues that didn’t resonate with underserved populations.
- Political Polling Challenges: During political elections, pollsters often struggle with non-response bias as specific demographic groups, notably young voters, are underrepresented. This has led to significant miscalculations of electoral outcomes in several instances, highlighting the critical need for effective engagement strategies.
- Social Media Impact: A survey distributed primarily through social media platforms faced immense selection bias as it only reached audiences engaged with those platforms. Thus, the results failed to capture the opinions of individuals who are less active online, leading to faulty conclusions on public sentiment.
To conclude about - survey biases
Survey biases are a critical obstacle in achieving valid and reliable research results. Awareness of the types of survey bias — such as selection bias, sampling bias, and response bias — is essential for researchers striving to enhance the quality of their data. By implementing comprehensive strategies to mitigate bias in surveys, researchers can optimize their methodologies and yield more accurate insights.
Furthermore, leveraging technology and adhering to best practices in survey design can yield more robust results while promoting a more inclusive and representative approach to data collection. As we navigate the complex landscape of survey biases, understanding these key concepts will empower researchers to make informed decisions and continue to refine their practices in an ever-evolving field.
Si quieres conocer otros artículos parecidos a Survey Biases: Key Types Every Researcher Should Know puedes visitar la categoría Surveys Sampling Methods.
Leave a Reply