What is the theory behind T-Test in survey analysis
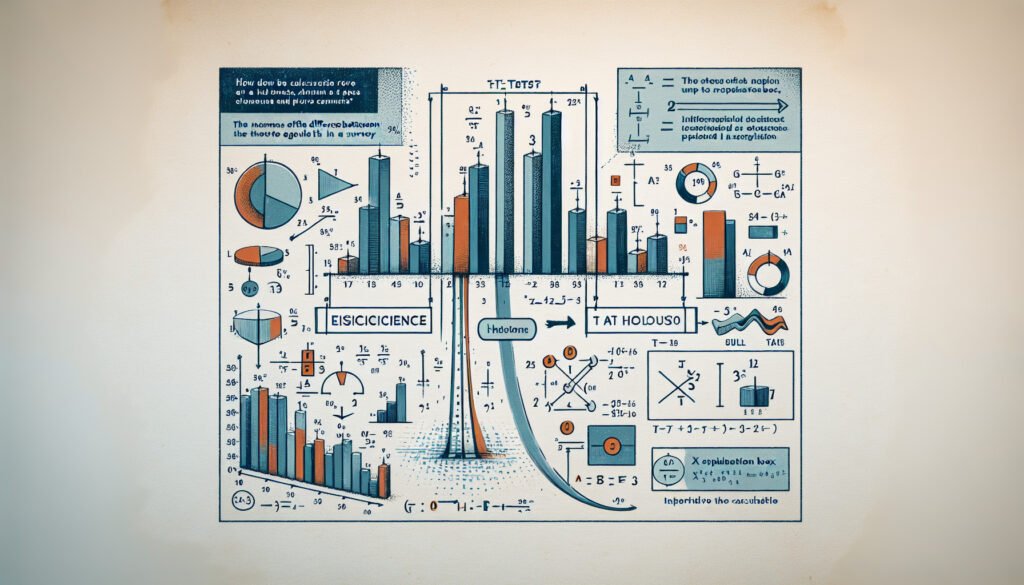
A t-test, also known as a t-analysis, is a vital statistical tool frequently utilized in survey analysis to assess whether the means of different groups differ significantly. By comparing the average outcomes of two or more samples or against a standard, researchers can evaluate hypotheses and derive meaningful insights. This methodology not only facilitates academic research but also finds relevance in various fields, including psychology, medicine, and social sciences.
The essential driving force behind a t-test is to identify the presence or absence of statistically significant differences while adhering to a structured hypothesis testing framework. When conducting a t-analysis, starting with a null hypothesis—designed to assert no difference between group means—helps establish a basis for argumentation. With an array of t-test variations, such as one-sample, two-sample, and paired t-tests, selecting the appropriate method is crucial to ensure valid conclusions from survey analysis.
-
Understanding the Theory Behind T-Test in Survey Analysis
- The Purpose of T-Tests in Statistical Analysis
- Key Concepts: Null Hypothesis and Alternative Hypothesis
- Types of T-Tests: One-Sample, Two-Sample, and Paired T-Tests
- Requirements for Conducting a T-Test
- The Origin of the T-Test: A Look at W. S. Gosset
- Essential Parameters for Performing a T-Test
- Interpreting T-Test Results: T-Value, Degrees of Freedom, and P-Value
- Common Applications of T-Tests in Survey Analysis
- Importance of Statistical Significance in Research Findings
Understanding the Theory Behind T-Test in Survey Analysis
The theory behind the t-test lies in its ability to evaluate the significance of differences between group means under the assumption of normality. This statistical method is grounded in inferential statistics, enabling researchers to make educated predictions about populations based on sample data. The t-test provides a loud voice to your data, enabling it to communicate insights that might not be immediately visible. Furthermore, understanding the intricacies of t-analysis helps researchers justify their findings convincingly, whether for academic publications, policy formation, or product development.
The Purpose of T-Tests in Statistical Analysis
The primary purpose of a t-test is to determine whether there is a statistically significant difference between the means of two groups or one group compared to a known standard. This analysis is crucial for validating research hypotheses and refining the decision-making process based on quantitative evidence. In survey analysis, the t-test assists in interpreting data collected from various groups or over different conditions, helping researchers identify trends and correlations.
Key Concepts: Null Hypothesis and Alternative Hypothesis
At the core of t-tests are two competing hypotheses. The null hypothesis (H0) asserts that there is no significant difference between the means being compared, essentially positing that any observed difference is due to sampling error. Conversely, the alternative hypothesis (H1) argues that there is a significant difference, suggesting that changes observed in sample means likely reflect actual differences in the population. Establishing these hypotheses is critical, as they guide the direction and interpretation of the t-analysis.
Types of T-Tests: One-Sample, Two-Sample, and Paired T-Tests
There are three primary types of t-tests, each designed for specific research needs:
- One-Sample T-Test: This test evaluates whether the mean of a single sample differs from a known value (usually a population mean).
- Two-Sample T-Test: Also known as an independent t-test, this assesses whether the means of two separate samples are significantly different from one another.
- Paired T-Test: This test is employed when the samples are related, such as before-and-after measurements on the same subjects, determining if there is a significant change.
Requirements for Conducting a T-Test
To conduct a valid t-test, certain assumptions must be met:
- The data should be measured at the interval or ratio scale level.
- For two-sample t-tests, the samples must be independent of each other.
- The data must approximately follow a normal distribution.
- The variances across the groups should be homogeneous or similar.
The Origin of the T-Test: A Look at W. S. Gosset
The development of the t-test can be traced back to William Sealy Gosset, a statistician who worked for Guinness Brewery in the early 20th century. In searching for a method to monitor the quality of beer with limited sample sizes, he devised what we now know as Student’s t-test. The name "Student" was a pseudonym he used to publish his findings, enabling him to maintain anonymity while working at the brewery. The significance of his contribution extends beyond beer production; it has become a foundational concept in statistical analysis, affecting various disciplines and research fields.
Essential Parameters for Performing a T-Test
To accurately perform a t-test, several key parameters must be sourced:
- Mean differences: The average values of the groups being compared.
- Standard deviations: Measures of the variability or dispersion of the sample data.
- Sample sizes: The number of observations collected in each group.
- Alpha level (α): A pre-determined threshold (commonly set at 0.05) for determining statistical significance.
- Critical value tables or software: Utilized for finding the threshold t-value that corresponds to the chosen alpha level and degrees of freedom.
Interpreting T-Test Results: T-Value, Degrees of Freedom, and P-Value
The outputs of a t-test provide essential information for validating hypotheses:
- T-Value: This statistic measures the size of the difference relative to the variation in the sample data. A large t-value generally indicates that the observed effect is unlikely to be due to chance.
- Degrees of Freedom: This parameter reflects the number of independent pieces of information available for estimating parameters, usually calculated as the total sample size minus one for each group.
- P-Value: This crucial value indicates the probability of obtaining a t-value at least as extreme as the observed one, assuming the null hypothesis is true. A low p-value (typically below the alpha level) leads to the rejection of the null hypothesis.
Common Applications of T-Tests in Survey Analysis
The t-test finds extensive applications in survey analysis, helping researchers make informed conclusions about their data. Some common applications include:
- Comparing average responses to survey questions across different demographics.
- Assessing the impact of experimental interventions, such as treatment vs. control groups in clinical trials.
- Evaluating changes in participant behavior or attitudes over time through longitudinal surveys.
- Testing differences in product satisfaction ratings before and after changes are implemented.
Importance of Statistical Significance in Research Findings
Statistical significance is a cornerstone of hypothesis testing and plays a critical role in the interpretation of research findings. It serves as the basis for determining whether the results observed in a study can be generalized to the larger population. By utilizing the t-test, researchers can ascertain the reliability of their discoveries and provide robust evidence to support their claims. This level of rigor is essential in academic research and practical applications alike, as it strengthens the validity of the conclusions drawn from survey analysis.
To conclude about - t analysis
In summary, the t-analysis, specifically through the implementation of t-tests, provides valuable insights that foster a deeper understanding of data trends and effects. By comprehensively grasping the theory behind this statistical method, researchers can effectively analyze survey data, validate hypotheses, and ensure their findings are robust and credible. As the importance of data-driven decision making continues to rise, mastering the t-test remains a critical skill for analysts across various fields.
Si quieres conocer otros artículos parecidos a What is the theory behind T-Test in survey analysis puedes visitar la categoría Surveys Sampling Methods.
Leave a Reply