Text Analysis: Definition, Benefits, and Examples Explained
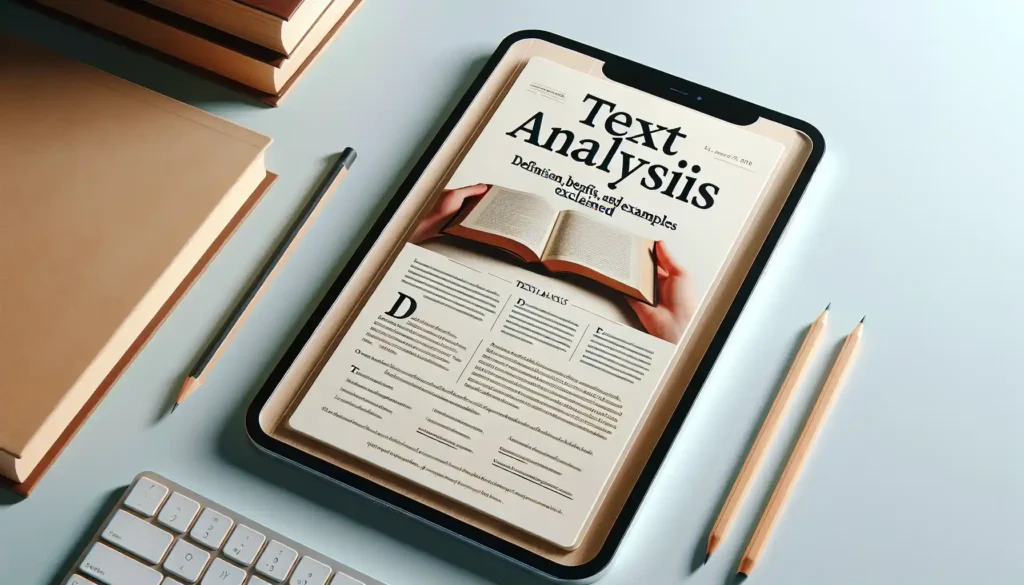
Text analysis is a powerful method employed by organizations to derive meaning from unstructured data. In today's fast-paced world, businesses are inundated with a vast array of textual information, including comments from social media, feedback from surveys, and emails from customers. By implementing text analytics, businesses can decode this unstructured content, turning it into structured data that reveals patterns and insights critical for decision-making. This methodology encompasses numerous techniques that not only enhance comprehension but also inform strategies that can lead to improved outcomes across various sectors.
The advent of technology has further amplified the significance of text analysis. Organizations now harness machine learning and natural language processing (NLP) to refine their analytics processes, allowing them to remain competitive in a data-driven landscape. By utilizing innovative tools like Qualtrics Text iQ, businesses can efficiently collect, analyze, and act upon feedback, leading to better customer and employee experiences. It’s clear that leveraging text analysis is no longer an option but a necessity for those seeking to thrive in the contemporary business environment.
- Understanding Text Analysis: Definition and Key Concepts
- The Importance of Text Analysis in Business
- Benefits of Implementing Text Analysis
- Key Techniques Used in Text Analysis
- Enhancing Text Analysis with Machine Learning and NLP
- Tools for Effective Text Analysis: A Closer Look at Qualtrics Text iQ
- Real-World Examples of Text Analysis in Action
- Challenges and Limitations of Text Analysis
- Future Trends in Text Analysis and its Applications
Understanding Text Analysis: Definition and Key Concepts
At its core, text analysis refers to the systematic computational procedure of evaluating text data to extract relevant information and insights. This technique encompasses a multitude of methods used to process text and make it interpretable. Key concepts include:
- Tokenization: The process of breaking down text into individual terms or tokens, facilitating further analysis.
- Named Entity Recognition (NER): Identifying proper nouns or specific entities, such as names, dates, and locations, within the text.
- Part-of-Speech Tagging: Assigning grammatical categories to each token, allowing for deeper understanding of text structure.
- Stemming and Lemmatization: Techniques for reducing words to their root forms, aiding in the standardization of text data.
These fundamental concepts pave the way for more advanced techniques in text analytics, expanding the utility of the insights obtained.
The Importance of Text Analysis in Business
In the business landscape, text analysis serves numerous vital functions that contribute to strategic decision-making. Understanding customer sentiment, gauging employee satisfaction, and identifying market trends are just a few areas where text analytics can make a significant impact. The overwhelming majority of feedback is provided in the form of text, rendering traditional quantitative methods insufficient for capturing the nuances inherent in this data. As such, organizations must look beyond numbers to fully comprehend needs and expectations.
Moreover, the insights derived from text analysis can lead to one of several outcomes, including:
- Enhanced Customer Understanding: Businesses can uncover genuine customer sentiments that are often concealed in raw comments.
- Improved Product Development: Analyzing user feedback can assist in tailoring products and services to better meet consumer demands.
- Effective Marketing Strategies: Insights from text analysis can refine messaging and targeting in campaigns for greater effectiveness.
- Risk Management: Early identification of potential issues through monitoring of sentiment can mitigate negative impacts.
Benefits of Implementing Text Analysis
Implementing text analytics provides numerous benefits that can drastically change how organizations operate and interact with stakeholders. Some notable advantages include:
- Data-Driven Decision Making: Organizations can leverage insights derived from text analysis to make informed strategic decisions based on real data rather than assumptions.
- Increased Efficiency: Automating the extraction of insights from large volumes of text saves considerable time and resources.
- Enhanced Customer Engagement: Understanding and responding to customer sentiments allows businesses to build relationships and foster loyalty.
- Competitive Advantage: Organizations employing advanced text analysis can respond to market shifts faster than those relying solely on traditional data analysis.
Key Techniques Used in Text Analysis
While various methods serve the purpose of text analysis, certain techniques stand out for their efficacy. These include:
Sentiment Analysis: Measuring Emotional Feedback
One of the most recognized techniques in text analytics is sentiment analysis, which assesses the emotional tone behind a series of words. This process enables businesses to categorize sentiments into positive, negative, or neutral. By understanding how customers feel about products, services, or experiences, organizations can tailor their approaches accordingly.
Methods for conducting sentiment analysis often incorporate machine learning models that are trained on extensive datasets, allowing for accurate predictions of sentiment. Some advanced software can even process sarcasm and context, making them particularly valuable.
Topic Detection: Identifying Relevant Themes
Another essential technique in text analysis is topic detection. This systematic approach categorizes text data into significant themes, helping businesses identify trending discussions or recurring issues within customer feedback. By using algorithms such as Latent Dirichlet Allocation (LDA), organizations can effortlessly pinpoint both prominent and emerging topics of interest.
Implementing topic detection facilitates targeted responses and streamlined decision-making processes, ensuring that organizations remain aligned with consumer needs.
Enhancing Text Analysis with Machine Learning and NLP
The integration of machine learning and natural language processing (NLP) significantly increases the capabilities and effectiveness of text analysis. Machine learning empowers algorithms to learn from data patterns, thereby enhancing accuracy over time. On the other hand, NLP facilitates the understanding of context, ensuring that analyses consider the nuances of human language, including slang and idioms.
Additionally, combining these technologies with traditional analytics provides a comprehensive framework for processing vast amounts of text data quickly. This synergy not only improves the reliability of insights but also allows for a more nuanced understanding of consumer behavior, ultimately leading to better strategic planning and decision-making.
Tools for Effective Text Analysis: A Closer Look at Qualtrics Text iQ
Among the myriad of available tools, Qualtrics Text iQ stands out for its robust text analysis capabilities. This platform combines text analytics with machine learning algorithms, providing businesses with the tools necessary to glean actionable insights from unstructured data. Key features of Qualtrics Text iQ include:
- Automated Insights: Quickly identifies sentiment and key themes from large datasets.
- Real-Time Analysis: Allows organizations to respond promptly to emerging issues.
- Customizable Dashboards: Provides tailored views that help stakeholders visualize data according to specific needs.
- Multi-Language Support: Extends functionality to organizations operating in diverse linguistic markets.
These features not only make text analysis more accessible but also empower organizations to transform insights into strategic actions effectively.
Real-World Examples of Text Analysis in Action
Text analysis has been successfully applied in various industries, showcasing its versatility and effectiveness. Here are a few compelling examples:
Healthcare
In healthcare, text analytics is utilized to mine patient feedback from surveys and online reviews, enabling hospitals to assess service quality and patient satisfaction. For instance, sentiment analysis on patient comments can reveal common concerns that hospitals need to address to improve overall care.
Retail
Retailers leverage text analysis tools to scan social media comments and reviews, allowing them to understand brand perception and consumer sentiment towards new product launches. By identifying trends and themes in customer feedback, retailers can adjust marketing strategies, ultimately driving sales and enhancing customer satisfaction.
Finance
In the financial sector, companies use text analysis to monitor customer feedback and sentiment with regards to their investment services. By utilizing tools to analyze textual data, they can identify potential risks and opportunities for growth, enabling proactive management of customer relationships.
Challenges and Limitations of Text Analysis
Despite its many advantages, text analysis does come with challenges and limitations that organizations must navigate. Notably:
- Data Quality: The efficacy of text analytics largely depends on the quality of the data collected. Inaccurate or poorly structured text data can lead to misleading results.
- Language Nuances: Variations in language, including slang, idioms, and regional dialects, can pose challenges in achieving accurate sentiment analyses.
- Complexity of Human Emotion: Understanding contextual emotions and sarcasm within text can be difficult, potentially leading to misinterpretations.
- Resource Intensive: Although tools like Qualtrics Text iQ streamline the process, effective text analysis can still require significant time and expertise to implement correctly.
Future Trends in Text Analysis and its Applications
As data continues to grow and evolve, so too will the text analysis landscape. Several emerging trends promise to shape the future of text analytics:
- Increased AI Integration: As artificial intelligence technology advances, it will likely enhance the capabilities of text analysis, leading to even more precise insights.
- Real-Time Analytics: The demand for immediate feedback in decision-making will push the development of more sophisticated real-time text analysis solutions.
- Expanded Use Cases: Industries beyond marketing and customer service are beginning to employ text analysis tools, such as human resources for sentiment analysis on employee engagement.
- Greater Emphasis on Ethics and Data Privacy: As data utilization increases, so will focus on ethical practices in data handling, necessitating transparent approaches to text analysis.
To conclude about - text analysis - text analytics
The significance of text analysis in today’s data-driven world cannot be overstated. Businesses that leverage text analytics can harness the wealth of unstructured data available to them, transforming it into actionable insights for enhanced decision-making. Despite the challenges that come with analyzing text, the benefits certainly outweigh these obstacles, particularly when armed with advanced tools and techniques. As technology continues to progress, the future of text analysis looks promising, offering organizations endless opportunities for growth, innovation, and improved customer experiences. It is evident that understanding the nuances of text analysis is crucial for any business aiming to thrive in this competitive landscape.
Si quieres conocer otros artículos parecidos a Text Analysis: Definition, Benefits, and Examples Explained puedes visitar la categoría Artificial Intelligence.
Leave a Reply