Sampling Methods: Types and Techniques Explained Clearly
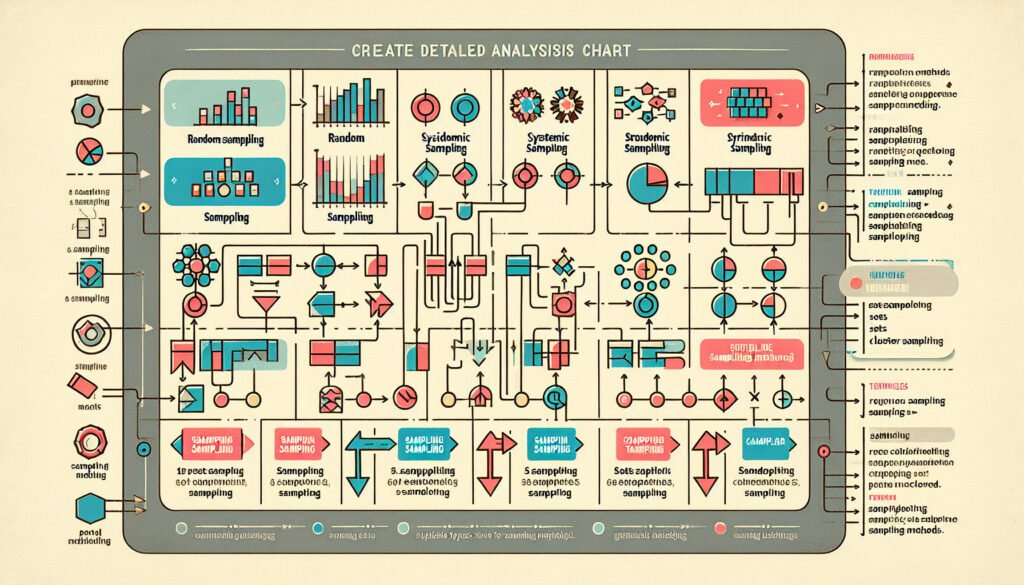
- Sampling Methods: Types and Techniques Explained Clearly
- Understanding Sampling Methods: The Importance of Representing Populations
- Defining Sampling Methods: An Overview of Key Concepts
- The Necessity of Sampling in Research: Why Every Detail Matters
- Differentiating Between Probability and Non-Probability Sampling
- Exploring Probability Sampling: Techniques for Minimizing Bias
- Non-Probability Sampling: When Randomness Takes a Backseat
- Factors Influencing the Choice of Sampling Method
- The Impact of Sampling on Research Findings and Insights
- Balancing Accuracy and Resource Limitations in Sampling
- Best Practices for Implementing Effective Sampling Techniques
Sampling Methods: Types and Techniques Explained Clearly
Sampling in survey research is an integral part of understanding how to gather data effectively. The method involves selecting a subset of a population to represent the entire group, making it crucial for conducting large-scale studies efficiently. Given the impracticality of surveying every individual in a large population, sampling methods allow researchers to gather data more cost-effectively and within a reasonable timeframe. Understanding the various sampling techniques is essential for ensuring that the data collected is both valid and reliable.
There are two primary categories of sampling: probability sampling and non-probability sampling. Probability sampling includes methods like simple random, systematic, stratified, and cluster sampling—where participants are randomly selected to minimize bias. Non-probability sampling, on the other hand, encompasses techniques such as convenience, quota, purposive, and snowball sampling, where selection is based on specific criteria or ease of access. Choosing the appropriate method of sampling depends on research goals, population characteristics, constraints, and the desired reach of findings. Despite the trade-offs in accuracy, effective sampling methodologies can lead researchers to uncover valuable insights while managing resource limitations.
Understanding Sampling Methods: The Importance of Representing Populations
Sampling methods play a critical role in the field of research, particularly when dealing with large populations. They are the backbone of data collection, allowing researchers to make inferences about a broader population without needing to collect data from every individual. This is vital for both practical and logistical reasons, as conducting a census can be time-consuming and costly.
Moreover, effective sampling is essential to represent the diversity of a population accurately. Poorly chosen samples can lead to biased results and may misrepresent the entire group, which can skew findings and conclusions. By understanding the different sampling types and examples, researchers can select appropriate methodologies that cater to their study's specific objectives, ultimately ensuring the validity of their results.
Defining Sampling Methods: An Overview of Key Concepts
To better understand sampling methodologies, it's important to define some key concepts associated with sampling. At its core, sampling is the process of selecting units from a population to make statistical inferences about that population. The selected units are referred to as the sample, while the complete group from which the sample is drawn is known as the population.
In sampling, researchers focus on minimizing sampling error—the difference between the sample statistic and the population parameter. Understanding different sampling methods allows researchers to mitigate these errors and derive conclusions that are more likely to reflect the true characteristics of the population.
The Necessity of Sampling in Research: Why Every Detail Matters
Sampling is not only necessary for operational reasons; it is also critical to the integrity of research findings. In many cases, researchers face constraints such as limited time, budget, or scope. Consequently, deploying effective sampling techniques in statistics examples becomes essential. By selecting representative samples, researchers can generalize findings to the larger population with a degree of confidence.
Consider, for example, a pharmaceutical company conducting trials for a new drug. It would not be feasible to test every potential patient. Instead, researchers must employ methods of sampling that yield reliable data while ensuring that the sample is adequately representative of the population targeted by the drug. This is an example of why every detail in the research design—including the sampling approach in research—matters significantly.
Differentiating Between Probability and Non-Probability Sampling
A fundamental distinction within sampling types revolves around whether they employ a random selection process. This division categorizes sampling into two broad types: probability sampling and non-probability sampling.
Probability Sampling
In probability sampling, each member of the population has a known and non-zero chance of being selected. This randomness reduces bias and enables the statistical extrapolation of results. Common types of sampling within this category include:
- Simple Random Sampling: Every member has an equal chance of being selected.
- Systematic Sampling: Selecting every nth individual from a list of the population.
- Stratified Sampling: Dividing the population into strata and sampling from each.
- Cluster Sampling: Randomly selecting entire groups or clusters of individuals.
These methods aim to minimize sampling bias and improve the representativeness of the sample.
Non-Probability Sampling
In contrast, non-probability sampling does not provide every individual in the population a chance of being selected. Researchers opt for this approach when convenience or specific criteria dictate the sample selection. Examples of this method include:
- Convenience Sampling: Selecting individuals who are easiest to access.
- Quota Sampling: Ensuring that certain characteristics are met within the sample.
- Purposive Sampling: Selecting individuals based on specific characteristics or purposes.
- Snowball Sampling: Existing study subjects recruit future subjects from among their acquaintances.
Non-probability sampling may introduce bias, but it can be useful in exploratory research or when quantitative methods are impractical.
Exploring Probability Sampling: Techniques for Minimizing Bias
Probability sampling is often favored in quantitative research because it helps minimize bias. There are several techniques, each with distinct advantages and ideal scenarios for use.
Simple Random Sampling: A Straightforward Approach
Simple random sampling is the most straightforward method where every member of the population has an equal chance of being selected. This method can be achieved using random number tables or software. While simple and effective for smaller populations, it can become cumbersome as population size increases.
Systematic Sampling: The Structured Selection Process
Systematic sampling offers a structured approach by selecting every nth individual from a population list. For example, researchers may determine their sample size and then select individuals at regular intervals. This ensures even coverage of the population but can introduce bias if there's an underlying pattern in the population list.
Stratified Sampling: Ensuring Representation Across Subgroups
Stratified sampling involves dividing the population into distinct subgroups or strata (based on characteristics like age, gender, income) and then selecting samples from each stratum. This ensures that important characteristics are represented, which is particularly useful for heterogeneous populations where certain subgroups might be underrepresented.
Cluster Sampling: Cost-Effective Group Selection
Cluster sampling is often more cost-effective, especially when dealing with large populations. Rather than sampling individuals, researchers randomly select entire clusters or groups. This is particularly useful when populations are widely dispersed geographically, allowing researchers to focus on specific areas rather than the entire population.
Non-Probability Sampling: When Randomness Takes a Backseat
While probability sampling is often preferred for its ability to reduce bias, there are scenarios where non-probability sampling fulfills research needs effectively. Understanding its sampling techniques can expand researchers’ toolkits.
Convenience Sampling: The Practical Choice
Convenience sampling involves selecting individuals who are easiest to reach, such as volunteers or those within proximity to the researcher. Though practical, it may lead to substantial bias if the chosen sample does not accurately reflect the broader population.
Quota Sampling: Meeting Specific Criteria
Quota sampling ensures that specific characteristics are represented within the sample. Researchers set quotas for certain demographic groups, ensuring a balanced representation within the limitations of each category. Like convenience sampling, this method prioritizes efficiency, but researchers must be conscious of potential biases.
Purposive Sampling: Intentional Selection for Targeted Insights
Purposive sampling is intentional and selective; researchers choose participants based on specific criteria relevant to the study. This approach is useful for qualitative research where insights from particular population segments are essential, but it may lack generalizability to the entire population.
Snowball sampling is often utilized in studies involving hard-to-reach populations. An initial subject is identified, and then they refer others, creating a "snowball" effect of sample gathering. This method can provide unique insights into hidden or marginalized populations but can also introduce bias as participants may share characteristics.
Factors Influencing the Choice of Sampling Method
The choice of a particular sampling method is influenced by various factors, including the research question, required level of accuracy, available resources, and the nature of the population. Researchers must carefully consider these aspects to select the most appropriate method of sampling for their study.
- Research Objectives: Determining what insights are desired from the study can guide sampling choices.
- Population Characteristics: Understanding the population's diversity informs the necessity for specific sampling techniques.
- Available Resources: Budgetary and time constraints often dictate which sampling methods are feasible.
- Desired Precision: Higher accuracy may require more sophisticated sampling methods to minimize error.
Recognizing these factors ensures that researchers employ the most fitting sampling methodologies for their objectives.
The Impact of Sampling on Research Findings and Insights
The sampling method has a significant impact on the validity and reliability of research findings. Poor sampling choices can lead to non-representative results, skewing conclusions and, ultimately, influencing decision-making. Sampling techniques in statistics examples illustrate the diverse implications of selection methods, from consumer behavior studies to clinical trials.
Having a representative sample fosters confidence in the results, which is essential for convincing stakeholders, policymakers, and academia of the findings' credibility. Therefore, researchers must ensure transparency regarding their sampling methodologies and justify their choices critically.
Balancing Accuracy and Resource Limitations in Sampling
While rigorous sampling methods are vital for accuracy, researchers often face resource limitations that necessitate compromises. Striking a balance between achieving representative samples and operating within limited resources is an ongoing challenge.
Analyses of sampling methods can provide insights into how researchers achieve this balance. This may involve utilizing a mix of probability and non-probability techniques or adopting more systematic approaches where feasible.
Best Practices for Implementing Effective Sampling Techniques
Conducting sampling effectively demands careful planning and execution. Here are some best practices for implementing effective sampling techniques:
- Define Target Population: Clearly identify the population to be studied.
- Choose Appropriate Method: Select sampling methods that best align with the research goals.
- Ensure Sample Size is Adequate: Calculate necessary sample sizes to ensure statistical significance.
- Document the Sampling Procedure: Keep detailed records of sampling processes for transparency.
- Evaluate Potential Bias: Assess methodologies to mitigate biases that may arise.
Adhering to these principles ensures comprehensive and representative data collection, leading to more reliable research outcomes.
To conclude about sampling methodology example, method for sampling, method of sample, methods of sampling, sampling methodologies, method sample, sampling techniques in statistics examples, types of sample, sampling types with examples, types of samples, type of sampling, type of sample, types of sampling, type of sampling statistics, kinds of sampling in statistics, types of sampling and examples, sampling types, sample types, types of samples statistics, types of sampling statistics, types of sampling stats, types of sampling in biostatistics, type sample, samples statistics, sampling statistics, sampling and statistics, sampling in statistics, sampling techniques example, sampling techniques, sampling technique, sample techniques examples, sampling techniques examples, technique of sampling, techniques of sampling, sampling technique examples, sampling method techniques, methods of sampling statistics, sampling techniques in statistics, sampling and sampling techniques, samples and sampling techniques, sampling and methods of sampling, sampling methods and techniques, sampling techniques and examples, sampling methods statistics, sampling and sampling methods, sample technique example, sampling techniques types, sampling method types, types of sampling methodology, type of sampling methods, type of sampling technique, types of sample methods, sampling methods types, sampling technique types, types sampling techniques, types sampling methods, type of sampling method, kinds of sampling method, types of sampling methodologies, different types of sample methods, types of sampling method, kinds of sampling methods, types of sampling methods, type of sampling techniques, types of sampling techniques, population sampling, sampling types in statistics, sampling approach in research, survey sampling, procedure for sampling, survey sampling methodology, sampling survey methods, sampling methods survey, survey sampling techniques, sampling survey method, sampling method example, example of sampling methods, sample method examples, sampling groups, sampling measurement.
Si quieres conocer otros artículos parecidos a Sampling Methods: Types and Techniques Explained Clearly puedes visitar la categoría Surveys Sampling Methods.
Leave a Reply